Data laying all around an enterprise’s premises and over the cloud is of no use unless it forms part of a bigger and clearer picture. This is what a data stack does by helping enterprises leverage data to its fullest potential- it turns raw data into insights that can be acted on and lead to business benefits.
The complicated modern enterprise of today cannot make do anymore with the obsolete ways of data management. The modern enterprise needs AIOps as a service and a modern data stack to power it.
Let’s begin with the basics and then look at how the modern data stack has evolved to become future-proof and potential-rich for today’s data-first enterprise.
The Basic Foundation of a Data Stack
A basic data stack can accomplish four foundational tasks:
- Data ingestion – It can move data from one place to another, particularly to process it.
- Data transformation – It can transform data into a form that can be utilized for processing.
- Data warehousing – It can store all data at one location, often on the cloud, for processing.
- Data analytics – It can process data to form insights through business intelligence and analytics.
The Cost of an Inefficient Data Stack
An inflexible, expensive setup – Companies using the traditional data stack often house it on-premise and are responsible for all costs associated with the infrastructure. They need everything from in-house data engineers to analysts to derive ROI from the humongous investment.
Since the setup is deeply connected and solidified, little changes can be made to it without risking breaking other parts of the system. In a fast-paced data environment, the traditional setup doesn’t cut it anymore.
Lesser use of data – As a company evolves, so do its data and computational power requirements. When it comes to scaling a traditional data center, the costs run high. Companies often need to choose between expensive analytics and cost-saving.
Data analysis needs computational power, which is not abundant in a traditional data system. Companies need to choose between running data pipelines and saving costs.
Delayed, expensive insights – Delayed insights are a waste. The traditional data stack performs slow ETL (Extract, Transform, Load) operations, delaying insights that are critical to obtain sooner rather than later. Consequently, business insights and decision-making lag.
Organizations miss critical opportunities and a competitive edge when insights aren’t available on time. Moreover, reports involve manual labor and are prone to errors, meaning professionals spend more time away from business-critical tasks.
The Modern Data Stack
Data ingestion – At this stage, data is consolidated from various sources, such as databases, server logs, edge/IoT applications, multi-cloud endpoints and third-party apps into a cohesive, single view and then returned to the client for analysis. AIOps meticulously solves volume and variety challenges associated with data by offering real-time analytics at the point of ingestion and enables analysis of stored data.
Data storage – A data warehouse or lake is often a cloud-based solution used to store all ingested data in one place. The data storage solution also acts as a query interface for data analytics and intelligence tools. Technologies such as security and data governance function in collaboration with data storage. It’s critical that the data storage solution support scalability, efficiency and granularity of computation, high availability and multiple data formats.
Data transformation – Once raw and unstructured data is moved into data storage, it needs transforming into user-friendly models. After transformation, it gets easier for data engineers and scientists to easily query data and extract insights from it to build dashboards and ML models. In modern systems, streaming data is transformed and enriched using feeds from contextual learning, PII, CVE, MITRE feeds and CMDB.
Data analytics and intelligence– Each customer environment is different. Analytics success depends on real-time, accurate and dynamic contextual intelligence. AIOps data stack enables context from cross-domain sources and lays down topological dependencies for correlation and root cause analysis. Modern log intelligence allows to experiment, automate and publish data and ML pipelines for observability. Explainable AI/ML pipelines help with dynamic baselining and anomaly detection.
Data governance – Data governance allows for data to be secure, accessible, available and conform to internal organizational standards as well as regulations. Modern AIOps data stack enables full-fidelity data copy for replay with UTM timestamps in an S3 bucket or Cloud Data Warehouse. Data can be stored in various formats for third-party analytics. And, noise reduction and compression can be useful along with versioning.
Before and After Modernizing your Data Stack
Research shows that over 60% of companies that embed data analytics with a differentiated user experience increase engagement and 57% of companies improve revenue. Additionally, 65% of surveyed product professionals agreed that analytics is the most critical component of the modern data stack and 73% said they planned to invest heavily in analytics tools.
Here’s what happens before and after modernizing your data stack-
- Before, getting huge quantities of varied data together presents a complex problem as traditional AIOps tools lack built-in capabilities, relying on third-party tools to do the same. After, with the modern AIOps data stack, varied data from events, tickets, metrics, logs and asset dependencies brings down the total cost of ownership of analytics and simplifies data analytics.
- Before, hybrid cloud environments failed to meet expectations due to their security vulnerability. After AIOps, efficient observability and data automation help address those challenges.
- Before, the usability of data remained restricted to a few use cases, such as events correlation. After the modern data stack, the ripples of log intelligence and business intelligence go far and wide in an organization. They help improve customer experience, boost revenue, mitigate risk, ensure compliance and security and reduce costs.
Learn more about CloudFabrix AIOps 2.0, Log Intelligence and Robotic Data Automation Fabric– the cutting-edge solutions for modern-day data management and analytics.
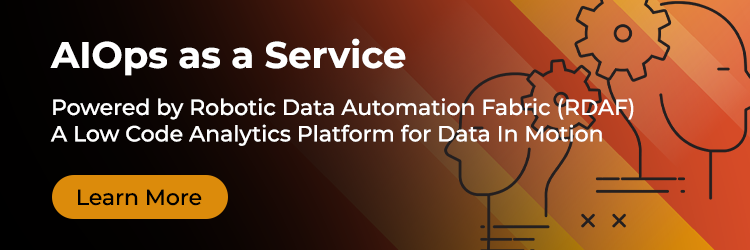