What is the need for observability in modern IT environments?
Enterprise data systems are like busy family households. You see a constant flow of activity to varying degrees from room to room. This activity includes people wandering, opening and closing doors. And then there are other streams constantly flowing through the household- electricity, water, Wi-Fi networks and more.
In modern enterprises, the data deluge is a critical issue. While we take the complexity for granted in a household, such is not allowed in a connected enterprise. In a family system, we get a grip over a few streams using intelligent systems such as thermostats, smart lighting and sensors.
However, in an enterprise, there are two ways of overseeing every activity:
- Monitoring – Monitoring solutions allow us to observe and locate what is happening in our IT systems. These systems act on predefined metrics and logs. Any abnormal activity in a log file would indicate an incident.
- Observability – Observability solutions allow IT teams to assess why changes occur in their systems. So, observability allows for asking questions that didn’t previously exist.
Observability is a trend only beginning to pick up. It’s so critical that by 2024, enterprises will increase observability tools adoption by 30%, according to Gartner. And, 90% of CIOs agree that observability is crucial to their success.
Data observability can help enterprises answer an urgent question- how healthy is your data? With differently formatted, diverse data flowing in and out of systems, several potential weaknesses could cause mission-crippling outages.
What are observability pipelines?
Before understanding observability pipelines, let’s shed light on first mile data. The first mile is about collecting and preparing data to be delivered to its destination for analysis. Several tasks constitute first-mile data operations, including data sanitization and context enrichment.
Observability pipelines create data hygiene. Data exploded over the last decade, and now with Kubernetes, customers want to spin up compute environments in seconds, multiplying applications, services and consequently, the data load.
A surge in the internet of things technology means several devices are now connected in various places, creating more data that requires monitoring and observing. An observability pipeline enables insight into whether the data is appropriately collected, why systems behave a certain way, and how the data flows through disparate systems.
Dimensions of observability pipelines include-
- Data collection – The capability to collect data (metrics, traces, logs) from a broad set of IT operations management tools.
- Context enrichment – The ability to inform incident management with data from additional sources such as CMDB, vendor systems, CI/CD tools, etc.
- Correlation & deduplication – The tasks that suppress noise and highlight actionable situations with real-time causality analysis.
- Data lineage and audit – The solution maintain an audit trail for data throughout it’s tenure from collection, shaping and routing to the target with the ability to play back the original data at any time
- Communication – Includes visual or textual communication around alerts and warnings.
How do observability pipelines help SREs / DevSecOps?
- Gain competitive advantage by leveraging diverse data
Modern IT environments are hybrid, containing a mix of modern and legacy tools. For IT leaders to leverage these tools and data and create an analytics-driven ecosystem, they need to effectively consume and integrate data from disparate systems with complex data models, APIs and archaic interfaces.
With observability pipelines, enterprises can automate data ingestion, preparation and integration to drive smart operations and gain real-time insights.
- Bridge talent gaps through low-code pipelines
It’s proven that the talent gap is a critical barrier to the successful adoption of AI and ML technologies. Observability pipelines using a low-code platform can be configured and authored by any IT enthusiast without specialized programming and data science knowledge.
Simple bots-based data pipelines can do so much more with minimal lines of code. RDAF by CloudFabrix allows data processing by bots (you get an extensive library of over 800 bots in over 20+ categories).
- Achieve hyper-automation
Present-day automation systems address domain-specific activities and fail to deliver end-to-end automation- creating silos. We need observability pipelines to automate decision-making across IT functions and make quality data available for AI/ML engines.
Organizations can transition from a loosely coupled set of automation tools and technologies to a connected hyper-automation with data pipelines.
- Leverage Edge and IoT
The boundaries of an IT environment are constantly blurring with the advent of the 5G technology and accelerated deployment of the IoT. There is an urgent need to analyze data near its source and compose distributed analytics from various domains.
Observability pipelines enable edge analytics with the bot network and data fabric. RDAF by CloudFabrix connects bots from multiple locations and sites to realize distributed analytics.
Observability pipelines help manage the data deluge, simplifying data collection, storage, transport and security and data processing across an enterprise.
Observability Pipelines in the context of AIOps
AIOps and observability work in tandem. AIOps acts as the brain behind the operations, leveraging past patterns found in data to develop future predictions and prescribe actions for the technology ecosystem to maintain its health.
AIOps triggers automation scripts and logic to mimic repetitive tasks in the technology ecosystem. Automation provides information for observability insights into real-time and historical behavior in the technology ecosystem and root-cause analysis. Observability, in turn, informs AIOps.
Robotic Data Automation Fabric offers a multi-tenant environment to design, explore, test, experiment, collaborate, reiterate, observe and deploy pipelines in production. RDAF comprises the following components:
- AIOps 2.0– Automating data and ML pipelines for observability and AIOps.
- RDA fabric – A secure and distributed databots network, data analytics fabric and orchestration.
- RDA app studio – A way to develop low-code data analytics applications.
DevOps and ML pipeline observability and automation is one of the critical use cases of an RDAF.
Move past traditional monitoring into observability pipelines and AIOps with CloudFabrix.
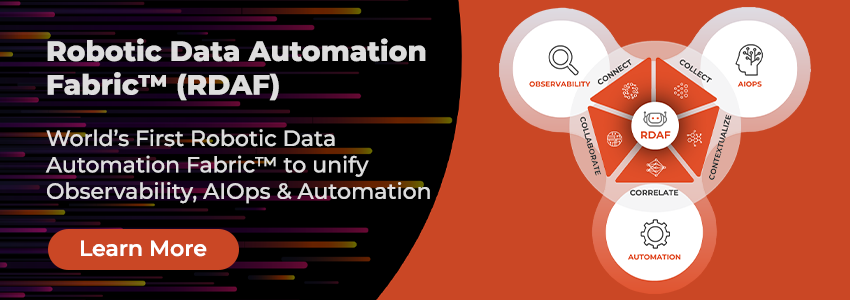