The traditional approach to operations management is quickly growing extinct in organizations, given the replacement of siloed architectures by integrated systems that can work with the multi-cloud, microservices, Kubernetes, and distributed architectures of the modern enterprise.
While the modernization of IT operations was already in full swing, the pandemic tipped it over. Through the pandemic, IT operations teams were on their toes and continue to be as organizations adopt hybrid work approaches.
Before we dive into how AIOps tools address operations challenges, let’s understand how legacy operations affect an organization’s growth, innovation, and success and why there came a need for AIOps.
Why is there a need for AIOps?
The traditional organization could make do with a physical, on-premise, mammoth-sized server and specialized professionals tasked with managing IT data and ensuring that an organization’s IT infrastructure ran smoothly.
Today, nearly every enterprise migrates applications and associated data to the cloud. Moreover, today’s applications are based on modern and distributed architectures that are not confined or defined by the physical limitations of an organization’s premises.
How does an organization stay compliant, highly available, secure, and resilient when it cannot physically contain or safeguard its IT data?
In today’s highly competitive era, organizations don’t just need to have the basics figured out but also optimize their efficiency and agility so that they can innovate fast.
However, challenges and complexity in a modern enterprise’s operations sit at either/all of three levels:
- Systems – Modular, distributed, and dynamic systems aren’t bounded by the physical premises of an organization.
- Data – The data each system generates constitutes the MELTs (metrics, events, logs, and traces). This data is high in volume, velocity, variety, and even redundant.
- Tools – The data deluge may be managed in a traditional organization through a host of rules-based tools that offer limited functionality with clunky integrations and lead to more blind spots in an IT infrastructure.
Cybersecurity risks plague organizations that still need to innovate and excel. Customer expectations continually rise as organizations strive to meet them.
Out go clunky, rigid, slow, and outdated IT operations methods, and in comes AIOps.
Definition of AIOps
According to Gartner’s 2021 Market Guide for AIOps Platforms, “over the past 12 months, AIOps formed part of the conversation in 40 percent of all inquiries with Gartner clients on IT performance analysis.”
And, AIOps continues its increasing proliferation and influence on the ITOM market with a market size projection of about $2.1 billion in 2025 with a CAGR of 19%, driven by digital transformation, a shift from reactive to a proactive approach and the need to make digital business observable.
Artificial Intelligence for IT Operations or AIOps involves AI and ML technologies along with big data, data integration, and automation technologies to make IT smarter and more predictive by complementing manual IT operations with machine-driven explainable decision-making.
AIOps employ data analytics, AI/ML, and big data capabilities to achieve the following:
- Collect and aggregate the deluge of data that modern IT systems produce through multiple IT infrastructure components, performance monitoring tools, and applications.
- Intelligently filter out the noise from the signals to identify significant patterns that link to system performance and IT availability.
- Diagnose root causes and either automate their resolution or route them to IT Ops teams for further evaluation and resolution.
AIOps value lies in consolidating fragmented IT operations tools with a single, intelligent, and automated AIOps tool, which accelerates response time, proactively manages IT, safeguards against outages and slowdowns, and frees up Ops teams to focus on the resolution of true anomalies instead of putting out false fires.
How does AIOps work?
Through algorithmic and automated analysis of all IT operations data and observability telemetry, AIOps assist SREs, DevOps, and IT Ops teams to work more efficiently. That means these teams detect IT issues earlier, resolve them quickly and ensure business continuity isn’t compromised.
With AIOps, teams can navigate the data deluge that comes with more and more distributed modern complex IT infrastructure without compromising security, compliance, and uptime.
As IT lies at the heart of any scale of digital transformation, AIOps enables organizations to go full-throttle instead of being held back by IT Ops at a time when organizations cannot afford to lag.
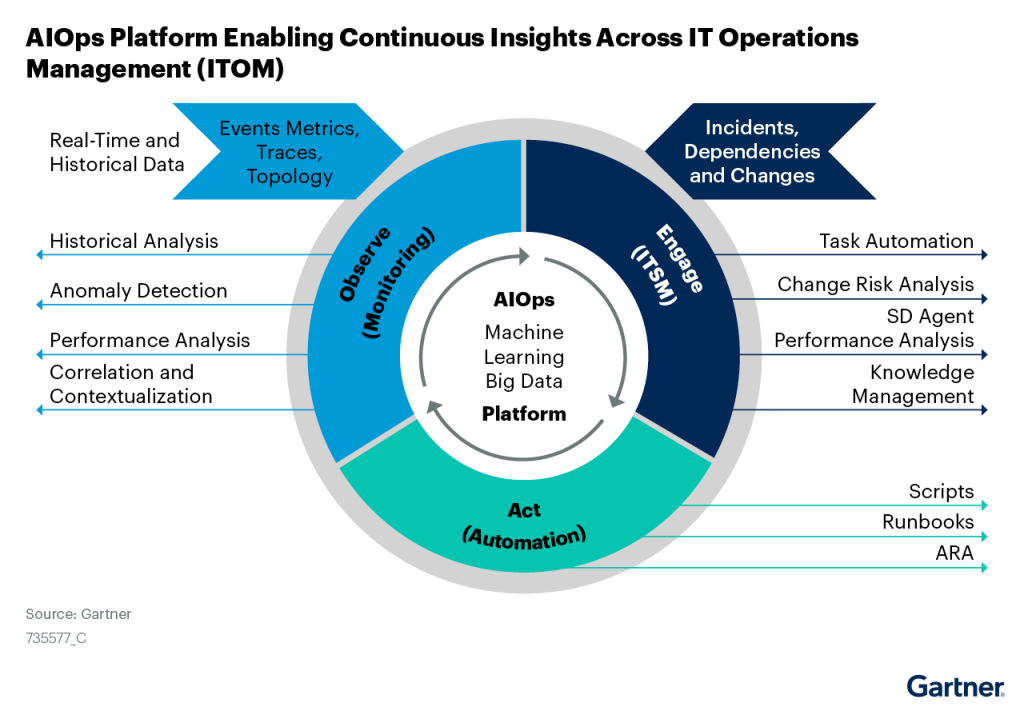
Major functions of AIOps in optimizing IT operations:
- Data ingestion – AIOps ingests, indexes, contextualizes, and normalizes the massive amount of redundant, noisy, and diverse data generated by a modern IT environment, selects data elements that might portray an anomaly, and filters out the vast majority of the noise. Modern AIOps tools support both real-time streaming data and historical data analysis.
- Data discovery – AIOps uses correlation to spot relationships and patterns in the selected, meaningful data and groups them to form advanced insights. Modern AIOps tools automatically discover IT assets and figure out the topology, indicating key information such as local dependencies and proximity in systems. As AIOps identifies how various IT assets support a business, it becomes easier to discover anomalies and act on them.
- Data inference – AIOps compresses and correlates events, builds the connection between topology and time to related events, and minimizes human intervention needed to infer data. Effective root cause analysis identifies the root of recurring issues so that decisions can be made automatically or using human intervention. Data enrichment and contextualization bring additional insight to raw data.
- Incidents management – AIOps notifies appropriate teams of anomalies and learns and improves on the job. It processes data from telemetry and events and predicts important incidents, continually learning event patterns.
- Autonomization – As data is collected and organized with context, decisions can be made based on real insights and accurate data. Actions can be automated to make recommendations and changes or send notifications to ecosystem components or users. Slowly, AIOps nudges an organization toward being an autonomous entity.
Types of AIOps Solutions
Gartner classifies AIOps solutions into two categories. Let’s see what those are.
- Domain-centric AIOps tools – These AIOps tools apply to a single domain, such as log monitoring, network monitoring, log collection, application monitoring, etc. These systems rely on their collectors to get “first-party” data. Often, when monitoring vendors tout themselves as AIOps, they are domain-centric, bringing AI to only the domains they manage. Even if these tools have started ingesting data from third-party systems, they tend to be costly, and many organizations limit the external data being ingested.
- Domain-agnostic AIOps tools – These AIOps tools function broadly and across domains such as monitoring, cloud, infrastructure, logging, etc., across third-party systems. They operate on humongous IT data ingested from IT infrastructure and systems across an organization and build models from this data (normalizing, enriching, and correlating them) to offer accurate inferences and decisions. Domain-agnostic AIOps tools are the future-proof solution for most modern organizations for their flexibility, accessibility, and agility.
Often, organizations only need one domain-agnostic AIOps solution to cover I&O, DevOps, SRE, and cybersecurity practices.
AIOps, ITSM, ITOM
ITSM or IT Service Management concerns how IT teams manage end-to-end IT service delivery, including designing, creating, delivering, and supporting IT services.
ITOM or IT Operations Management concerns with managing the provisioning, capacity, performance, and availability of networking, computing, and application resources and the overall efficiency, quality, and experience of delivery.
As ITSM and ITOM overlap in organizations, ML and analytics can become enablers of that convergence. An AIOps strategy, as per Gartner, observes, engages, and acts efficiently. Newer use cases can be envisioned and implemented across ITSM and ITOM for automated event remediation, incident management, intelligent ticketing, and routing which means that AIOps can lead to proactive service resolution.
Gartner’s 2021 Market Guide for AIOps Platforms observes that many ITSM vendors have now included AIOps capabilities by investing in internal development or partnering with AIOps platform vendors.
“AI-powered ITSM enables effectiveness, efficiency and error reduction for infrastructure and operations (I&O) staff by applying context, advise, actions and interfaces of AI on ITSM tools.”
However, Gartner warns organizations to beware of tools that offer only basic search-and-display capability and tout it as AIOps.
Related Reading: AIOps and Observability- Which One Should Enterprises Focus on First?
What are the key AIOps use cases?
Here are the critical use cases of AIOps.
Alert noise reduction
Over 40% of organizations get bogged down by over a million alerts every day, and 11% receive over 10 million daily alerts. Consequently, organizations require more streamlined alert noise reduction to make sense of this data.
AIOps help accomplish this by correlating multiple alerts into a single entity and reducing redundancy, offering AI/ML-powered recommendations using algorithms that spot patterns and show related alerts, and forecasting to reduce alert volume through suppression, grouping, and prevention.
With static threshold baselining, organizations can only create alerts per pre-established levels, which doesn’t work in today’s dynamic environment. Modern AIOps solutions address this through granular controls to tune telemetry collection intervals and dynamic thresholds that establish a baseline for every metric and raise alerts when deviations occur.
Incident room
Incident management with AIOps employs AI/ML recommendations to put incidents together and identify their root cause quickly. Incidents are logged based on the root cause and the resolution. Then, concerned teams are notified via various channels, such as Slack, Teams, or email.
AIOps allows Ops teams to create exceptions to solve an incident so that policies exist for the next time. IT personnel can then respond to incidents using resolution recommendations from AI/ML and perform root cause analysis to prevent repetition.
Then, incidents are resolved and closed. AIOps improves critical metrics such as Mean Time to Resolution, which creates a ripple effect throughout the organization.
Predictive analytics
Modern AIOps solutions can convert unstructured data such as logs/events/alerts into time-series data to run predictive analytics. Though the model can run on any time-series data, it may not be feasible to do this across all data points in a state of data deluge. So, organizations can employ the following process to identify key data points by correlating high-level KPIs with IT metrics.
- AIOps can learn about the various management relationships (assets monitored by monitoring tools), metrics/logs/traces available for each asset, and the relationship between assets (infrastructure/applications).
- AIOps tools can identify the key KPIs and assets that need to be continuously monitored to eliminate critical incidents. Critical assets can be learned through a top-down approach where users input the KPIs or by analyzing historical data.
- Then, AIOps can find metrics/logs/traces- through ML algorithms- that have a high correlation to the health and performance of critical assets.
- AIOps can then continuously monitor the identified critical observability data to detect anomalies adjusted for the seasonality behavior and to predict anomalies and alerts to initiate preventive measures.
Asset intelligence
Real-time asset intelligence is a critical component of AIOps unless you have a log of all your assets and how they are connected. Such correlation is not possible in complex IT infrastructure.
Real-time asset intelligence enables IT monitoring with rich information that builds context and directs Ops teams to issues in servers, networks, topologies, apps, storage, and services. It helps with risk and compliance management by ensuring IT asset information is always up-to-date and readily available for compliance purposes.
IT asset intelligence brings IT changes and their potential impact to light and provides a 360-degree view of asset inventory, lifecycle, performance, utilization, dependencies, and compliance. Finally, IT asset management allows for dependency mapping, which is a critical cornerstone of dependency mapping.
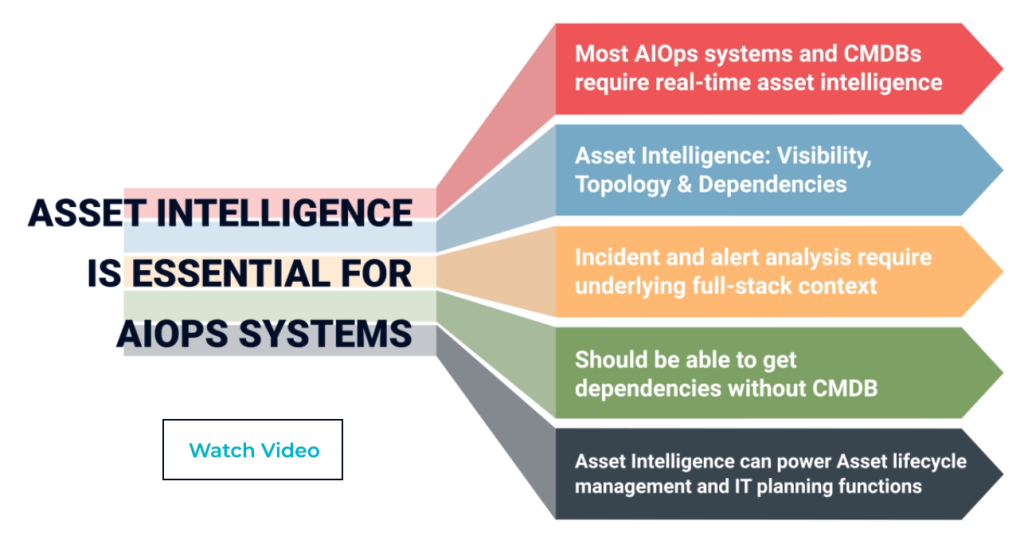
Log intelligence
A modern log intelligence feature can perform the following-
- Log reduction, routing, replay – Log correlation and noise reduction can save organizations costs. It can provide full-fidelity data, log archival and log replay from particular timestamps.
- Log enrichment – To achieve quick discovery and resolution times for anomalies, organizations need to enrich logs data and trim the noise to add context to real-time streaming data.
- Log EdgeAI – Organizations that employ edge computing need to optimize log ingestion at the edge and apply NLP to make the data verbose to discover anomalies and patterns in log data at the edge. Data pipelines at the edge cut down on edge-to-cloud costs and boost productivity.
Related Reading: Observability Pipelines and AIOps Can Make IT Smarter
Who is using AIOps and Why?
Here are a few entities leveraging AIOps globally in all industries and markets.
Enterprises with complex IT environments
Organizations with extensive IT environments that face complexity and scalability issues adopt AIOps to support the IT function so that it, in turn, supports business goals. As these organizations adopt more modern and sophisticated technologies, AIOps helps them achieve transformation while ensuring customer experience throughout.
Multi-cloud SMEs
AIOps is also embraced by small and medium enterprises that use multi-cloud environments and need to develop and release software products faster. AIOps allows SRE teams within SMEs to offer better digital services with each upgradation without compromising on the quality of deliverables for customers and worrying about malfunctions, glitches and outages.
DevOps teams in all enterprises
As modern enterprises employ DevOps methodologies, change becomes continuous, and you need to know where to look when incidents happen. AIOps can shorten the time required to diagnose incidents and direct teams where something needs fixing.
As AIOps gives operations teams visibility into when and where developers make changes, CI/CD cycles can run uninterrupted, and software delivery be accelerated. Moreover, as DevOps pipelines generate huge amounts of data, AIOps can analyze it quickly and continuously to recommend proactive actions and data-driven decisions.
Organizations with hybrid environments
Organizations benefit from moving workloads to the cloud but may still want to maintain certain applications on-premises. Hybrid environments bring a set of operational IT challenges.
AIOps can offer a holistic and thorough view of the organization’s IT infrastructure on-premises and in the cloud and help Ops teams understand dependencies and relationships between the two as they change dynamically, offering much-needed service assurance.
Businesses prioritizing digital transformation
Digitization of business processes makes an organization future-proof, efficient, agile, and competitive. IT lies at the core of digital transformation and can make or break these changes. Automating ITOps through AI means preventing glitches, reducing response times, proactively building IT resilience, and keeping outages at bay.
AIOps for Various Stakeholders and Teams

AIOps is adopted and leveraged by various teams, such as DevOps, SRE, ITOps, cybersecurity, and business leaders. Put another way, AIOps has far-reaching consequences and impact all business and IT areas.
- DevOps – Metrics, traces, and log analysis are primary functions for DevOps teams. As DevOps matures as a practice, AIOps use cases focus on production metrics such as user engagement, quality, and business relevance. AIOps can ingest data across IT systems and offer product and platform views to DevOps teams.
- ITOps – IT operations teams begin the journey with event correlation and broaden into metrics and logs analysis and behavioral analytics with primary goals of anomaly detection, diagnostics, and root cause analysis. Finally, ITOps teams can benefit from automating actions through integrations, scripts, and automated workflows.
- SRE – SRE objectives resemble those of ITOps and DevOps. However, event correlation and log analytics aren’t primary objectives of SRE teams, but the analysis of those informs actions toward resilience. AIOps platforms offer real-time topology and dependency insights, making analyses easy for SRE teams.
- Business teams – Business leaders and teams concern themselves with efficiency, user engagement, productivity, and behavior analysis to deliver better business decisions faster. Modern AIOps focuses not only on quantitative IT metrics but on qualitative KPIs such as efficiency and productivity of people, processes, and technology.
Related Reading: Data Value Gap – Data Observability and Data Fabric- Missing Piece of AI/AIOps
What to look for in an AIOps solution?
Gartner advises organizations to juxtapose AIOps tools by looking for the following AIOps attributes:
Explainable AI
Many IT operations staff aren’t comfortable with AI due to past experiences of AI running amok, causing false alarms or simply being mysterious. To earn the trust of Ops teams, AI must be explainable and transparent within an AIOps tool.
Users must have visibility into how models are created and decisions made within the purview of AI. AIOps vendors are only starting to integrate explainable and interpretable AI within their AIOps tools. A majority of AIOps tools in the market function with a black-box approach to AI that fails to build trust in AI in Ops teams and leads to more resistance to adoption.
Data ingestion and handling
AIOps platforms must be capable of analyzing data at rest, meaning historical data and data in motion, meaning real-time streaming data to be future-proof. The modern AIOps tools will allow ingestion, indexing, and storage of MELTs.
These tools will have edge AI capabilities to analyze data directly at the point of ingestion, in real-time, without the need to save it into a database. Modern AIOps will also provide correlated analysis across multiple streams of real-time and historical data.
ML and AI analytics
AIOps platforms will use the following analytical approaches to support the ops teams of today and tomorrow.
- Statistical, probabilistic analysis leverages correlation, clustering, classifying, and extrapolation methods on metrics across the IT environment.
- Automated pattern discovery and prediction discover patterns in historical and/or streaming data to predict anomalies along with varying degrees of probability.
- Anomaly detection employs patterns discovered through the previous analytical methods to determine baseline normal behavior and report departures from the dynamic baseline as anomalies. Anomalies must also be correlated with business impact and change management to provide more contextual information.
- Probable cause determination prunes down the correlations established through pattern discovery to identify causality chains that link cause and effect.
- The topological analysis offers contextualized analysis by deriving patterns from data within a topology, establishing relevance, and illustrating dependencies.
- Adaptive prescriptive solutions are aimed at resolving any issue detected. These suggestions are based on a database of historical solutions to recurring issues or determined via crowdsourcing. Over time, AIOps can identify the most relevant solution after assessing various possibilities.
Automated Insights
Automated insights from AIOps reduce the visual overload faced by IT Ops teams by highlighting interesting data instead of having teams find a needle in a haystack.
As AI analyzes signals, identifies areas for human intervention, and communicates so using effective means such as visual interfaces, notifications and collaborative tools, IT Ops teams gain the bandwidth to step back from the day-to-day and work toward IT resilience.
Adaptive remediation
The true value of AI and ML isn’t in rule-based solutions but in AIOps tools that identify the most appropriate action from many possible ones for an issue at hand. Choosing a prescriptive approach doesn’t take sophisticated AI algorithms. But the complex modern IT environment needs better, such as adaptive remediation and the potential of true AI.
Where does the AIOps market stand today?
According to Gartner, the AIOps market is expected to grow at 15% YoY between 2020 and 2025.
As per Insight Partners, the AIOps market size will grow from $2.83 billion in 2021 to $19.92 billion by 2028 at a CAGR of 32.2%.
Global Market Research insights reveal that the AIOps market size exceeded $2 billion in 2020 and is expected to grow over 20% from 2021 to 2027 to hit a whopping $10 billion.
MarketsandMarkets estimates that the global AIOps platform market size will grow from $2.55 billion in 2018 to $11.02 billion in 2023 at a CAGR of 34% during the forecast duration.
According to Allied Market Research, the global AIOps market size stood at $26.33 billion in 2020 and is now expected to reach $644.96 billion by 2030 at a CAGR of 37.90% between 2021 and 2030. “It has become essential for monitoring and managing modern IT environments that are hybrid, dynamic, distributed and componentized.”
Related Reading: New Modern Data Stack for AIOps as a Service
What are the benefits of implementing AIOps?
AIOps unlocks the following benefits for organizations, helping them achieve operational and business success.
- Higher employee productivity and customer satisfaction.
- More efficient use of IT infrastructure and capacity.
- ML-powered algorithms on huge volumes of data to garner insights and take action.
- Data integrity while collecting data in multiple formats from different sources.
- Reducing downtime costs with predictive analytics and preventing and resolving issues before they impact business.
- Lesser firefighting and costly outages.
- Faster time to deliver new software services and products.
- Aligned business outcomes with IT services.
- Better correlation between change and performance.
- Higher efficiency in managing change.
- Better employee experience for ITOps teams as AI takes on bulky, monotonous tasks.
- Reduction in false alarms and quicker root cause analysis with AI algorithms.
- Gain a unified, streamlined, real-time view of the IT environment.
- Unlock the real value of data by doing away with siloed responses.
- Support traditional IT infrastructure, public cloud, private cloud, hybrid cloud, edge AI applications and microservices and Kubernetes architectures.
- Keep cybersecurity incidents at bay with resilient IT infrastructure.
- Maintain compliance with ease with all data in one place.
- Reduce costs by reducing the headcount of operations teams by bringing in automation and AI.
- Spend less time troubleshooting and more time innovating solutions to support customers or internal staff with digital transformation initiatives.
Related Reading: The Future of AIOps
The Cost Impact of AIOps
When assessing the cost impact of AIOps on an organization, we advise leaders to look beyond the technology’s ability to reduce costs and toward both direct and future potential benefits to the business.
AIOps enhances flexibility, reduces risk, prevents disruptions in critical IT assets, and accelerates detection and resolution of anomalies. It’s critical to account for these qualitative benefits when considering the cost vs. benefits of AIOps.
From that perspective, AIOps optimizes revenue generation, boosts customer satisfaction and retention, protects brand reputation, and directly and indirectly impacts business performance and the bottom line.
Download the white paper “The Economic Impact of CloudFabrix AIOps” for an in-depth cost impact consideration.
Related Reading: Democratizing Data Using a DataFabric & How it Benefits IT Enterprises
About CloudFabrix AIOps
CloudFabrix’s Datacentric AIOps unifies observability, security, and automation with Robotic Data Automation Fabric and brings edge, data center and multi-cloud IT infrastructure under one purview of IT.
Read more here about the far-fetched ripple effects of employing AIOps in your organization.
Download our white paper “Accelerate your Digital Transformation Journey with AIOps”.