What is AIOps
AIOps or Artificial Intelligence for IT Operations refers to a set of technologies that augment human decisions with autonomous decisions driven by AI and machine learning that learn patterns, relationships from data.
AIOps is the term originally coined by Gartner, and pictorially illustrated in the following way

Why AIOps is Needed?
Emerging digital IT paradigm shifts like Hybrid IT, Multi-Cloud, Microservices & Containerization, Serverless, Software-Defined Datacenter, etc. are creating compelling new opportunities for IT leaders. However, these same paradigm shifts have also led to an increase in monitored assets, diverse operational tools, and exponential growth of operational data, leading to petabytes in a single day. IT operations personnel are having to deal with many different tools and data sources and often struggle to make swift operational decisions with the amount of noise in the data.
As a result, modern IT organizations are facing challenges in many areas including:
- Unable to make sense of large volumes of data (metrics, logs)
- Constantly increasing Alert and Event Noise
- Complex and Lengthy IT Problem Resolution Process
- Unable to predict/prevent IT service degradations or outages
- Unable to predict capacity issues – More …
- Unending IT complexity
Machine learning is the technique that is squarely designed to process such high volumes of IT data and automate decision-making. Machine learning can augment human understanding in processing large and complex datasets that are typical in IT operations. With rapid advancements in AI and Machine Learning technology and the widespread availability & affordability of high-performance compute (CPU/GPU), enterprise leaders are beginning to take big bets on AI, to succeed in their digital and cloud transformation initiatives
How AIOps can Help IT Organizations
AIOps technologies can help improve IT service reliability, improve customer experience, reduce unplanned outages and improve SLA. IT organizations can realize significant operational efficiencies, reduce costs and make staff more productive by complementing traditional operations with machine learning-driven decisions.
For example, AIOps can help the number of IT tickets that a service desk or NOC team needs to handle, and AIOps achieves this by eliminating duplicate alerts or simply grouping multiple related problems together to create meaningful and actionable tickets. Similarly, AIOps can help point to the root cause when complex IT problems occur, or AIOps can even provide some early signals or predictive insights to prevent problems before they start impacting customers.
Type of AIOps Vendors and AIOps Platforms
AI and ML can be applied at various places in an IT domain. AIOps vendors can generally be categorized as domain-centric and domain-agnostic, as described by Gartner
Domain-centric AIOps vendors are focused on bringing AI-driven decisions to a certain domain – typically in the monitoring spaces – like Application Monitoring, Infrastructure Monitoring, Network monitoring, etc.
Domain-agnostic AIOps vendors are generally focused on cross-domain IT data, bringing data from ITOM, ITSM, CMDB/ITAM tools and providing aggregate intelligence, cross-domain correlation, bringing context to data, and driving broader autonomous decisions at scale.
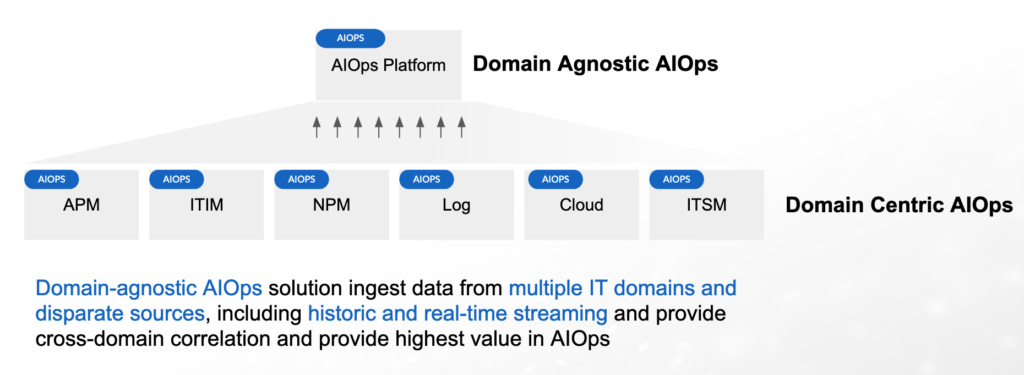
Navigating the AIOps Landscape
One of the challenges for customers is to navigate and understand the AIOps landscape because most vendors do claim AIOps, but the application of AIOps is different. Following are some common themes I have seen:
- 1) Monitoring-centric AIOps(Domain-Centric)
- Observability i.e monitoring tool vendors are now claiming AIOps, but this is localized or domain-centric application of AI. This kind of AIOps might be sufficient if your entire IT estate is being monitored by one or two monitoring tools. However, for large enterprises this is rarely the case. Some large IT organizations have at witnessed least 15+ tools in healthcare, pharma and financial industries.
- Sample vendors: AppDynamics, Dynatrace, NewRelic, Datadog, LogicMonitor, ScienceLogic etc.
- 2) ITSM centric-AIOps (Domain-Centric)
- Vendors who are originally focused on incident management, have primarily event and incident data, and are now beginning to apply AI/ML to incident specific use cases. For example, these vendors can help predict assignment group based on learning from previous incidents, or classify incidents based on incident text description. However, this is form of AIOps is again, localized to Incidents, which generally are reactive in nature, and cater to service-desk, NOC and ITOps personnel.
- Some vendors have expanded into IT operations space just because of thier sheer footprint and sometimes customers may feel it is better to go with existing vendor because of easier access to data, but this may not be optimal in the long run.
- Sample vendors: ServiceNow, PagerDuty, Cherwell …
- 3) Data-Lake centric-AIOps (Domain-Centric)
- These type of vendors are primarily known for their capabilities to serve as a massive data store or a data-lake for log data. However, these vendors later expanded to store more types of data i.e time series metrics data, configuration data and more. These vendors started to provide AI/ML on the data they have, primarily around predicting some patterns and providing good visualization and anlaytics on wealth of data they own, but one major gap in these kind of AIOps is they have very little understanding and context of the application stack, topology, serviceability, supportability and how apps are tied to a business or service.
- Sample vendors: Splunk, Elastic, Graylog …
- 4) Pure play AIOps: (Domain-Agnostic)
- These vendors are truly domain-agnostic, operating on IT data from all domains (apps, microservices, infra, incidents, cloud …) and provide aggregate intelligence and augmented decisions taking into consideration a very wide spectrum of IT data, thus yielding better results than purely domain-centric platforms. One major advantage of such platforms is also the notion of understanding the application and business context that allows for driving better ML decisions and reducing false positives and unintended consequences that may be prevalent in machine driven decisions.
- Sample vendors: CloudFabrix, BigPanda, Moogsoft …
AIOps is new and navigating the landscape can be challenging. However, we @ CloudFabrix helped many customers navigate through these challenges and successfully implemented AIOps. To learn more or Contact CloudFabrix